
Artificial Intelligence (AI) rapidly transforms how we predict, manage, and respond to natural disasters, including wildfires. With advanced capabilities to process massive datasets and generate predictions, AI promises a new era of efficiency and accuracy in emergency warnings.
However, recent incidents in America have raised critical questions about the reliability of these systems in high-stakes scenarios. AI's failure to detect and issue timely fire warnings has sparked debates over its limitations and implications for public safety.
This article delves into the shortcomings, causes, and potential solutions for AI in emergency response systems.
The Promise of AI in Emergency Management
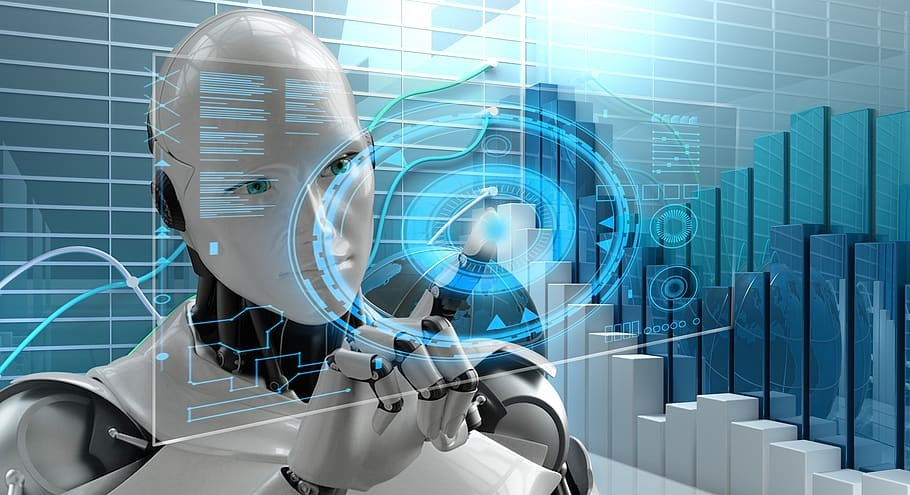
AI’s application in emergency warnings is rooted in its ability to analyze vast amounts of data from sensors, satellite images, weather reports, and historical records. For wildfire detection specifically, AI leverages machine learning (ML) models to:
Identify Early Signs: Detect heat signatures and smoke patterns in satellite imagery.
Predict Fire Spread: Simulate the trajectory of fires based on environmental factors like wind speed, vegetation, and humidity.
Support Resource Allocation: Recommend optimal deployment of firefighting resources.
Improve Communication: Automate alerts and updates to relevant authorities and communities.
Organizations like the National Interagency Fire Center (NIFC) and startups like Descartes Labs have utilized AI to monitor wildfire activity. Despite these advancements, there are instances where AI has faltered, leaving communities vulnerable.
Recent Failures of AI in Fire Warnings

Several notable cases in America illustrate the pitfalls of relying solely on AI for emergency alerts:
California Wildfires (2023): AI-based systems failed to issue timely alerts for fires in certain rural regions, resulting in significant property damage and loss of life.
Oregon’s Holiday Farm Fire (2020): Predictive models underestimated the fire’s rapid spread, leaving firefighters ill-prepared to respond effectively.
Hawaii Wildfire Crisis (2023): AI tools failed to predict the intensity of the fires, which were exacerbated by high winds and dry conditions, delaying evacuation notices.
In these instances, the reliance on AI systems revealed several flaws, including data limitations, algorithmic biases, and a lack of human oversight.
Why Did AI Fail?
Data Gaps: The quality and quantity of data significantly impact AI’s performance. Sparse or outdated datasets can lead to inaccurate predictions. For example, rural areas may lack sufficient sensors, leading to incomplete analysis.
Complex Environmental Factors: Fires are influenced by unpredictable variables like wind shifts or sudden lightning storms. AI struggles to adapt to such dynamic changes in real time.
Algorithmic Bias: AI models trained on specific datasets may not generalize well to diverse terrains and conditions, such as urban versus forested environments.
Over-Reliance on Automation: In some cases, insufficient human intervention exacerbated the delays in recognizing system errors or overrides.
Latency in Decision-Making: AI-generated alerts often need validation, creating time lags that can be critical during emergencies.
Consequences of AI Failures in Fire Warnings

The consequences of these failures extend far beyond technical errors, directly affecting lives, property, and ecosystems:
Loss of Life: Delayed evacuations or warnings can result in preventable fatalities.
Economic Damage: Fires can destroy homes, businesses, and infrastructure, leading to billions in losses.
Erosion of Trust: Communities lose faith in technological systems, making future warnings less effective.
Environmental Impact: Unchecked fires devastate wildlife and vegetation, contributing to long-term ecological imbalances.
Policy and Legal Repercussions: Failures often lead to public inquiries and lawsuits, pushing authorities to reassess the reliability of AI systems.
Balancing AI and Human Expertise

To mitigate these failures, a balanced approach that combines AI with human
oversight is essential. Key strategies include:
Improved Data Collection: Expanding sensor networks and incorporating real-time data from diverse sources like drones, satellites, and citizen reports.
Hybrid Systems: Integrating AI-driven analysis with human judgment to validate and act on alerts. For instance, trained meteorologists or fire management experts could cross-check AI predictions.
Algorithm Enhancements: Updating machine learning models to factor in complex and dynamic variables, improving their adaptability.
Public Education: Raising awareness about the limitations and strengths of AI systems ensures communities understand when to act on warnings.
Investment in R&D: Government and private sector collaboration can enhance AI capabilities by funding research into more robust algorithms and data infrastructure.
Success Stories: How AI Works When Done Right

Despite the challenges, several success stories highlight AI’s potential when implemented correctly:
California’s ALERTWildfire: A camera-based AI network that monitors fire-prone areas and successfully detects multiple wildfires early in their development.
Australia’s Bushfire Prediction Models: AI tools have accurately predicted fire paths, enabling timely evacuations and resource deployment.
NASA’s FIRMS (Fire Information for Resource Management System): Integrating AI with satellite data to provide global fire detection and monitoring.
These examples showcase how combining robust AI systems with reliable data and human oversight can lead to effective disaster management.
The Path Forward: Building Trust in AI Systems
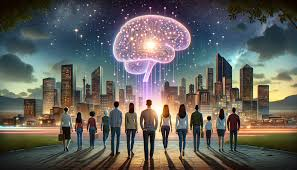
To restore trust in AI for emergency warnings, stakeholders must address its shortcomings while emphasizing its strengths:
Transparency: Clear communication about how AI models operate, including their limitations, fosters confidence among users.
Accountability: Establishing protocols to review AI failures and applying lessons learned to prevent future issues.
Collaboration: Building partnerships between technology developers, government agencies, and emergency responders ensures comprehensive solutions.
Scalability: Adapting AI systems to function effectively across diverse geographical and environmental conditions.
Ethical Standards: Ensuring AI systems prioritize safety and equity, minimizing biases that may neglect underserved communities.
Conclusion
While AI offers unprecedented opportunities to revolutionize fire detection and emergency management, its failures underscore the importance of continuous refinement and human involvement.
By addressing data gaps, improving algorithms, and fostering collaboration, AI can become a reliable ally in mitigating disasters. Building trust requires transparency, accountability, and a commitment to learning from past missteps.
As technology advances, a balanced approach integrating AI’s strengths with human expertise will ensure that communities remain prepared and protected in the face of natural disasters like wildfires.
Hozzászólások