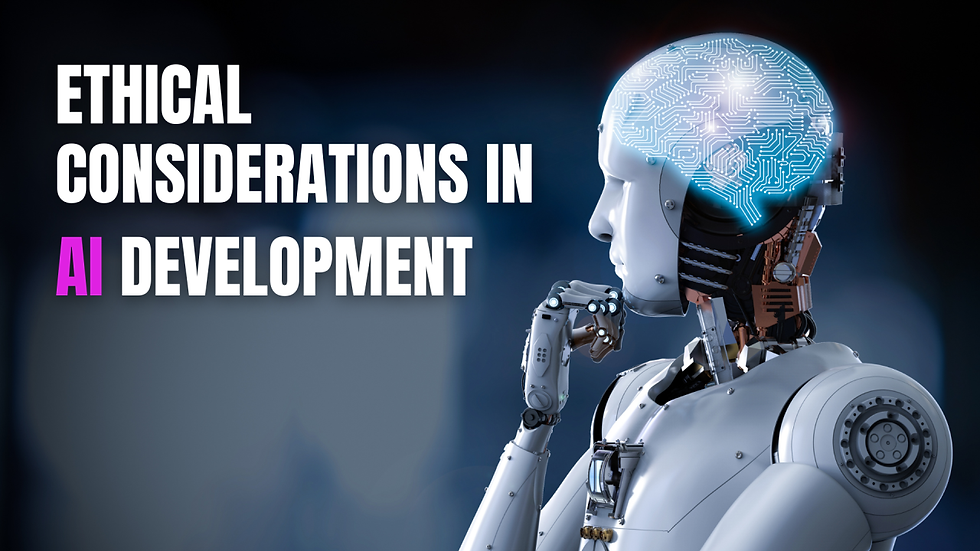
Artificial Intelligence (AI) has transitioned from a futuristic concept into a powerful force shaping industries, governance, and daily life. AI technologies have demonstrated transformative potential, from chatbots and virtual assistants to predictive analytics in healthcare and autonomous vehicles. However, as AI applications proliferate, the ethical dilemmas and biases ingrained in these systems have garnered increasing scrutiny.
The Promise of AI
AI systems are designed to learn from data and make decisions or predictions that would typically require human intelligence. Their promise lies in their ability to process vast datasets, identify patterns, and produce outcomes faster and often more accurately than humans. In domains such as healthcare, AI algorithms detect diseases early, and in finance, they analyze markets for smarter investments.
Despite this promise, the neutrality of AI is increasingly questioned. Instead of being purely rational, AI systems often reflect their creators' biases, ethics, and decisions.
The Foundations of AI Ethics
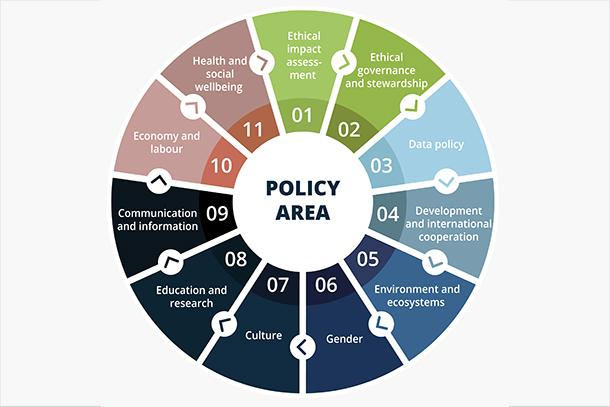
AI ethics is an interdisciplinary field exploring the moral implications of AI technologies. Ethical AI aims to ensure that these systems:
Operate Transparently: The decision-making processes of AI should be explainable and understandable.
Promote Fairness: AI should avoid biases and ensure equitable outcomes for all user demographics.
Enhance Human Well-being: Technologies should benefit humanity without causing harm.
Be Accountable: Systems must allow for auditing and remediation if outcomes are harmful or discriminatory.
Key Principles of Ethical AI
Accountability: Organizations deploying AI must take responsibility for their systems' actions and decisions.
Bias Reduction: Efforts should be made to identify and mitigate biases in datasets and algorithms.
Privacy Preservation: Personal data used in AI systems must be safeguarded.
Transparency: AI should be open about its methods, limitations, and objectives.
Decoding Bias in AI
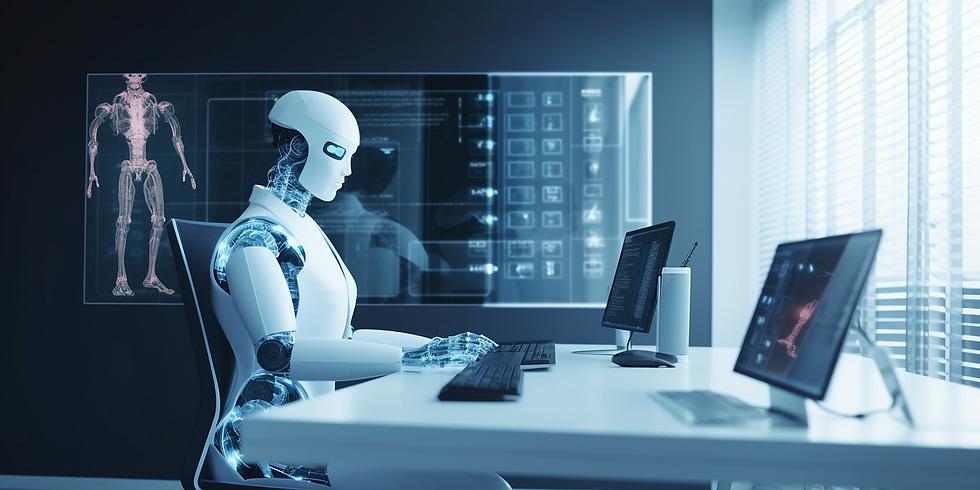
Bias in AI arises when an algorithm produces results that are systematically prejudiced due to faulty data or design. These biases can perpetuate or even exacerbate existing societal inequities.
Types of Bias in AI
Data Bias: When training data reflects societal stereotypes or historical inequalities, AI models perpetuate these biases. For example, facial recognition systems trained predominantly on lighter-skinned faces often misidentify individuals with darker skin tones.
Algorithmic Bias: The underlying design or architecture of algorithms can inadvertently prioritize certain groups or outcomes over others.
User Interaction Bias: If AI learns continuously from user behavior, it might amplify harmful stereotypes. For instance, search engine algorithms may reflect and reinforce gender or racial biases in search results.
Automation Bias: Humans tend to over-rely on AI outputs, assuming they are impartial, which can magnify errors if the system is biased.
Examples of AI Bias in Action
Criminal Justice: Predictive policing tools have been shown to target minority communities disproportionately, as historical arrest data used for training often reflects systemic biases in law enforcement.
Hiring Algorithms: Some companies have discovered that their AI recruiting tools penalized resumes with women’s names or references to female-oriented activities due to historical patterns in hiring data.
Healthcare: AI algorithms predicting medical costs have sometimes underestimated the needs of minority patients, reflecting systemic disparities in access to care.
Facial Recognition: Numerous studies highlight inaccuracies in recognizing people of certain racial groups, raising concerns about its use in surveillance and identification.
The Path to Ethical AI
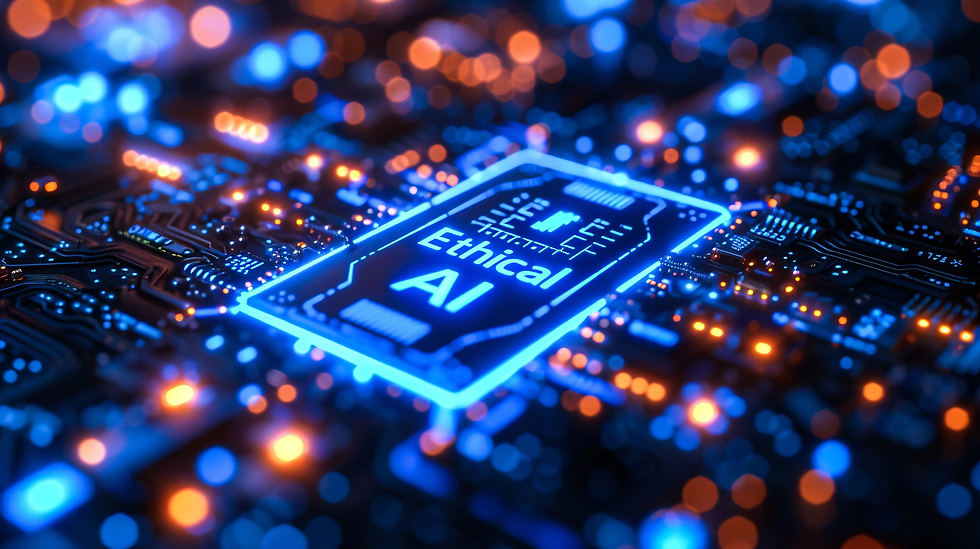
Bias Identification and Mitigation
Diverse Datasets: Ensure datasets used for training AI models are representative of the population.
Regular Audits: Organizations should frequently audit AI systems to identify and address biases.
Inclusive Development Teams: Building diverse teams to design and test AI reduces the likelihood of blind spots.
Regulations and Standards
Governments and industry bodies worldwide are beginning to set guidelines for ethical AI development:
EU's AI Act: Proposes classifications of AI systems based on risk and enforces transparency, accountability, and safety.
AI Bill of Rights (USA): Outlines key protections to prevent harm from AI technologies.
Explainability and Interpretability
Black-box AI models whose decision-making processes are opaque—raise significant concerns. Researchers are focusing on explainable AI (XAI) to improve interpretability, enabling stakeholders to trust and validate AI systems.
Building Accountability Mechanisms
Ethics Boards: Companies like Google and Microsoft have established AI ethics boards to oversee projects.
Impact Assessments: Evaluating the social and environmental consequences of AI deployment is becoming a standard practice.
The Moral Debate: Can AI Be Truly Ethical?
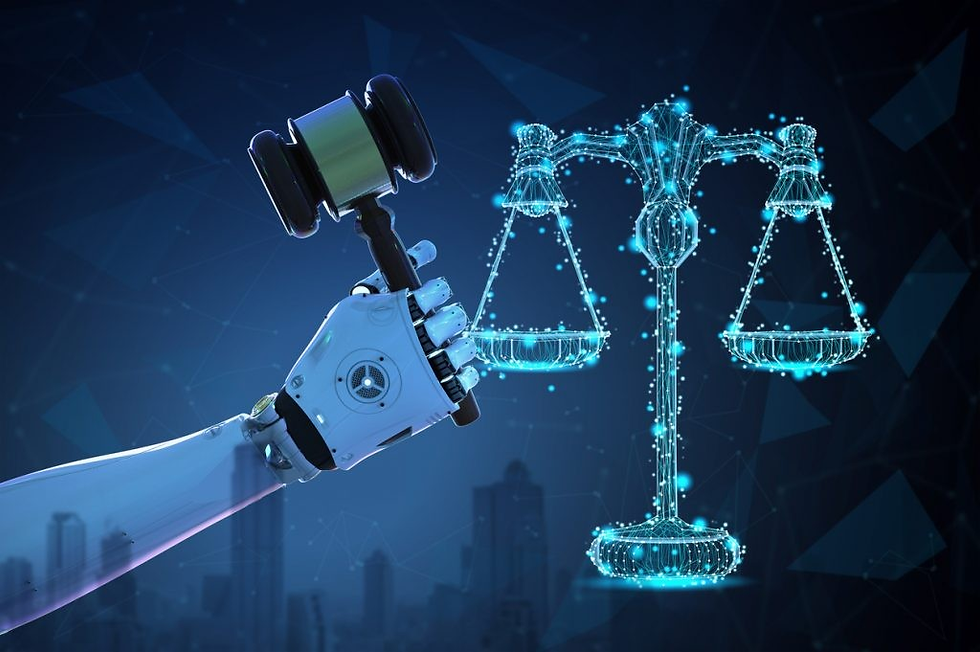
Critics argue that achieving truly ethical AI is inherently paradoxical:
Subjective Ethics: What’s ethical in one culture may not be considered the same in another. Designing a universally “ethical” AI might be unrealistic.
Human Bias in Design: Since AI reflects its creators, ethical shortcomings may always persist.
Profit-Driven Motives: Many AI systems are developed by corporations prioritizing profitability, which can overshadow ethical considerations.
Future Directions and Solutions
Collaboration Between Stakeholders
Multidisciplinary Teams: Ethicists, technologists, policymakers, and community representatives must collaborate to shape AI frameworks.
Global Policies: Given AI’s borderless nature, international cooperation on guidelines and enforcement is crucial.
Advancing Technical Solutions
Fairness Metrics: Researchers are developing quantifiable measures to evaluate fairness in AI outcomes.
Federated Learning: Allows AI models to train on diverse datasets without compromising individual privacy.
Promoting AI Literacy
Educating the public about how AI systems function, their benefits, and potential pitfalls empowers users to demand ethical practices.
Conclusion
AI holds immense potential to improve lives, but it must be wielded responsibly. Ethical challenges surrounding AI bias are not insurmountable but require deliberate, collaborative, and sustained efforts to resolve.
While achieving “truly ethical” AI may remain aspirational, transparency, accountability, and fairness provide a guiding framework for building trust in these technologies.
As we navigate the complex intersection of innovation and ethics, one thing is clear: the quest for ethical AI is as much about shaping our societal values as it is about technological advancement.
Kommentare